Bryan Wang
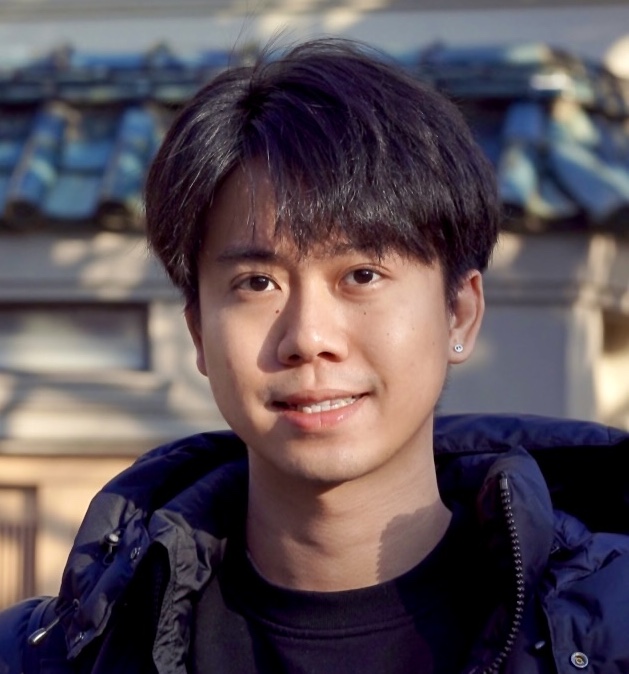
đ§ bryanw@dgp.toronto.edu
đ Google Scholar
đŠ Twitter
Research Interests:
- Human-AI Interaction
- Creativity Support
- Generative AI
I am a Computer Science Ph.D. student at the University of Toronto, working with Prof. Tovi Grossman at the Dynamic Graphics Project Lab.
My research area focuses on Human-AI Interaction.I apply my expertise in human-computer interaction and machine learning to develop interactive AI systems and study their implications for users. These systems leverage state-of-the-art AI to enhance human capabilities in creating and consuming digital content, including video, audio, images, and GUIs. Recently, my exploration has centered on leveraging multimodal generative AI models to enable novel interaction paradigms.
I graduated with a Bachelorâs degree in computer science from National Taiwan University. I have also spent wonderful time as a research intern at Google, Adobe, and Meta.
I run pro bono office hours for HCI. If youâre a junior student seeking advice or guidance in HCI research, Iâm here to help.
News
Mar 18, 2024 | Attended IUI to present LAVE! See more details on the project website where I discussed several future directions for agent-assisted video editing! |
---|---|
Dec 14, 2023 | Two papers accepted to IUI â24! One paper from my Meta internship on LLM-Augmented Video Editing. The other, led by MSc student Stephen Brade from U of T, on AI-assisted synthesizer sound retrieval and exploration. More details to come. |
Oct 26, 2023 | One paper on AI-assisted synthesizer sound retrieval and exploration was accepted to the Machine Learning for Creativity and Design Workshop at NeurIPS â23. Great Work led by MSc student Stephen Brade! |
Oct 6, 2023 | One paper on LLM agent accepted to EMNLP â23 Findings Track. Congrats to the co-authors at Google! Details to come. |
Aug 15, 2023 | Thesis proposal âDemocratizing Content Creation and Consumption through Human-AI Co-Pilot Systemsâ is accepted to UIST 2023 Doctoral Symposium. Looking forward to the event! |
Aug 3, 2023 | Promptify is accepted to UIST 2023. Great work led by mentee Stephen Brade! |
Aug 1, 2023 | Co-chairing the Student Innovation Competition at UIST 2023 on LLM systems. Submit your idea! |
Jul 28, 2023 | The ICML AI and HCI workshop was a great success. A big shout out to all co-organizers, speakers, reviewers, and authors! |
Jun 2, 2023 | Invited talk at UW HCI Seminar and Huawei Canada. |
May 15, 2023 | Started internship at Meta Reality Labs â Research with Raj Sodhi in Hrvoje Benkoâs group. |
Apr 12, 2023 | Invited talk at Meta Reality Labâs HCI seminar. |
Mar 31, 2023 | Excited to serve on the program committee of UIST 2023. |
Jan 13, 2023 | Three papers accepted to CHI 2023. Congrats to all co-authors! |
Jan 12, 2023 | Gave a talk at Takeo Igarashiâs Lab at Univeristy of Tokyo. |
Oct 30, 2022 | Presented paper Record Once, Post Everywhere: Automatic Shortening of Audio Stories for Social Media at UIST 2022 in Bend, Oregon. |
Oct 27, 2022 | Project page for Record Once, Post Everywhere (ROPE) is up! |
May 31, 2022 | Started an in-person summer internship with Yang Li at Google Research in Mountain View, CA. |
May 9, 2022 | Wrapped up a remote internship with Gautham J. Mysore and Zeyu Jin at Adobe Research. |
Representative Publications
-
Enabling Conversational Interaction with Mobile UI using Large Language ModelsIn Proceedings of the 2023 CHI Conference on Human Factors in Computing Systems (CHI) 2023
-
Screen2Words: Automatic Mobile UI Summarization with Multimodal LearningIn Proceedings of the 34th Annual ACM Symposium on User Interface Software and Technology (UIST), 2021
-
Soloist: Generating Mixed-Initiative Tutorials from Existing Guitar Instructional Videos Through Audio ProcessingIn Proceedings of the 2021 CHI Conference on Human Factors in Computing Systems (CHI), 2021